We are currently developing hierarchical Bayesian techniques to infer the unknown coefficients in initial-boundary value problems (IBVPs) for linear parabolic partial differential equations.
Finitely many noisy measurements of the solution field are made available, at a sequence of time points, in the interior of a domain of interest and for the boundaries. A main novelty of our approach to solve such class of inverse problems relies on the assumption that the boundary parameters are unknown and modeled by means of adequate probability distributions. Using the linearity of the equation, we marginalize the contribution of the boundary parameters from their joint law with the unknown equation coefficients, deriving the exact expression of the likelihood function of the coefficients that is associated with the forward problem.
As an example, we provide a full implementation of the IBVP for the one-dimensional heat equation, showing how to infer the thermal diffusivity parameter, which is modeled a priori by means of a space-dependent stationary lognormal random field. Expected information gains and predictive posterior densities for observable quantities are also numerically estimated for different experimental setups.
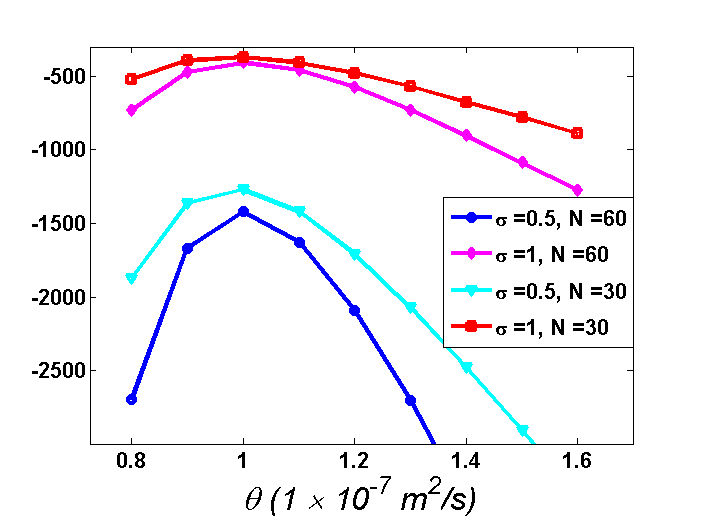
Non-normalized log-posterior distributions for the thermal diffusivity θ using different number of observations N
and different values of the measurement error standard deviation σ.
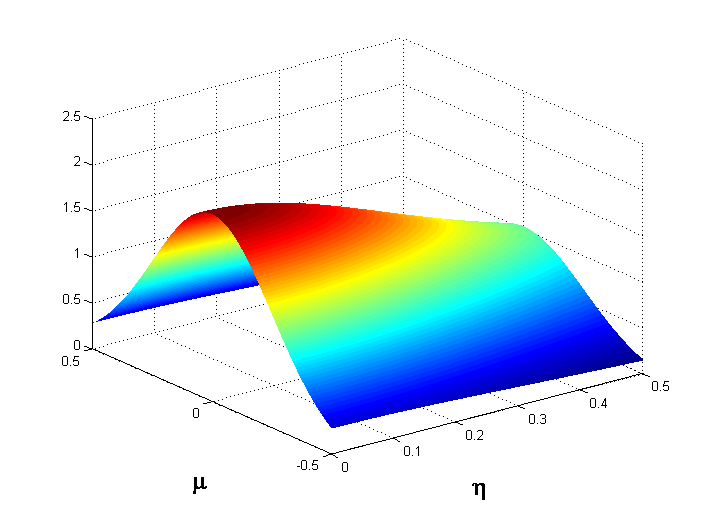
Joint prior density for the hyperparameters μ and η,
with μ ̴̴ N(0, 0.25) and η ̴̴ half-Cauchy(0.5).
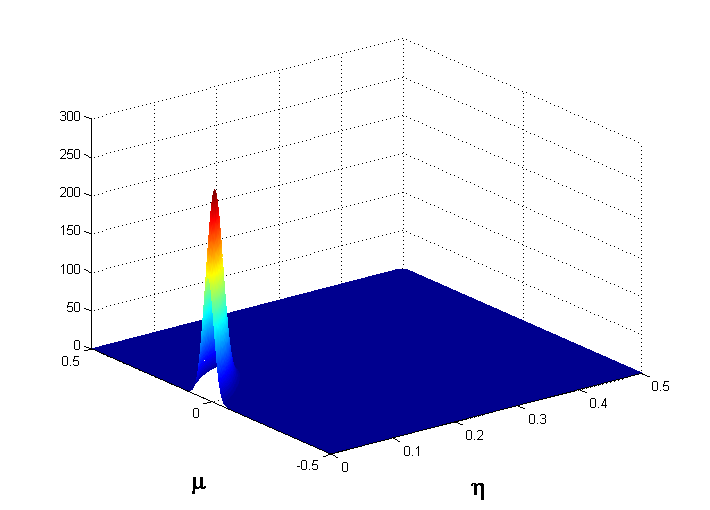
Laplace’s approximation for the posterior density
of the hyperparameters μ and η.