In this work, we present an extension of the forward-reverse algorithm by Bayer and Schoenmakers [Annals of Applied Probability, 24(5):1994--2032, October 2014] to the context of stochastic reaction networks (SRNs).
It makes the approximation of expected values of functionals of bridges for this type of processes computationally feasible.
We then apply this bridge-generation technique to the statistical inference problem of approximating the reaction coefficients based on discretely observed data. To this end, we introduce a two-phase iterative inference method in which, during the first phase, we solve a set of deterministic optimization problems where the SRNs are replaced by their reaction-rate ODE approximation; then, during the second phase, the Monte Carlo version of the Expectation-Maximization (EM) algorithm is applied starting from the output of the previous phase.
By selecting a set of over-dispersed seeds as initial points for the phase I, the output of parallel runs of our two-phase method is a cluster of maximum likelihood estimates. For convergence assessment we use techniques from the theory of Markov chain Monte Carlo.
Our results are illustrated by numerical examples.
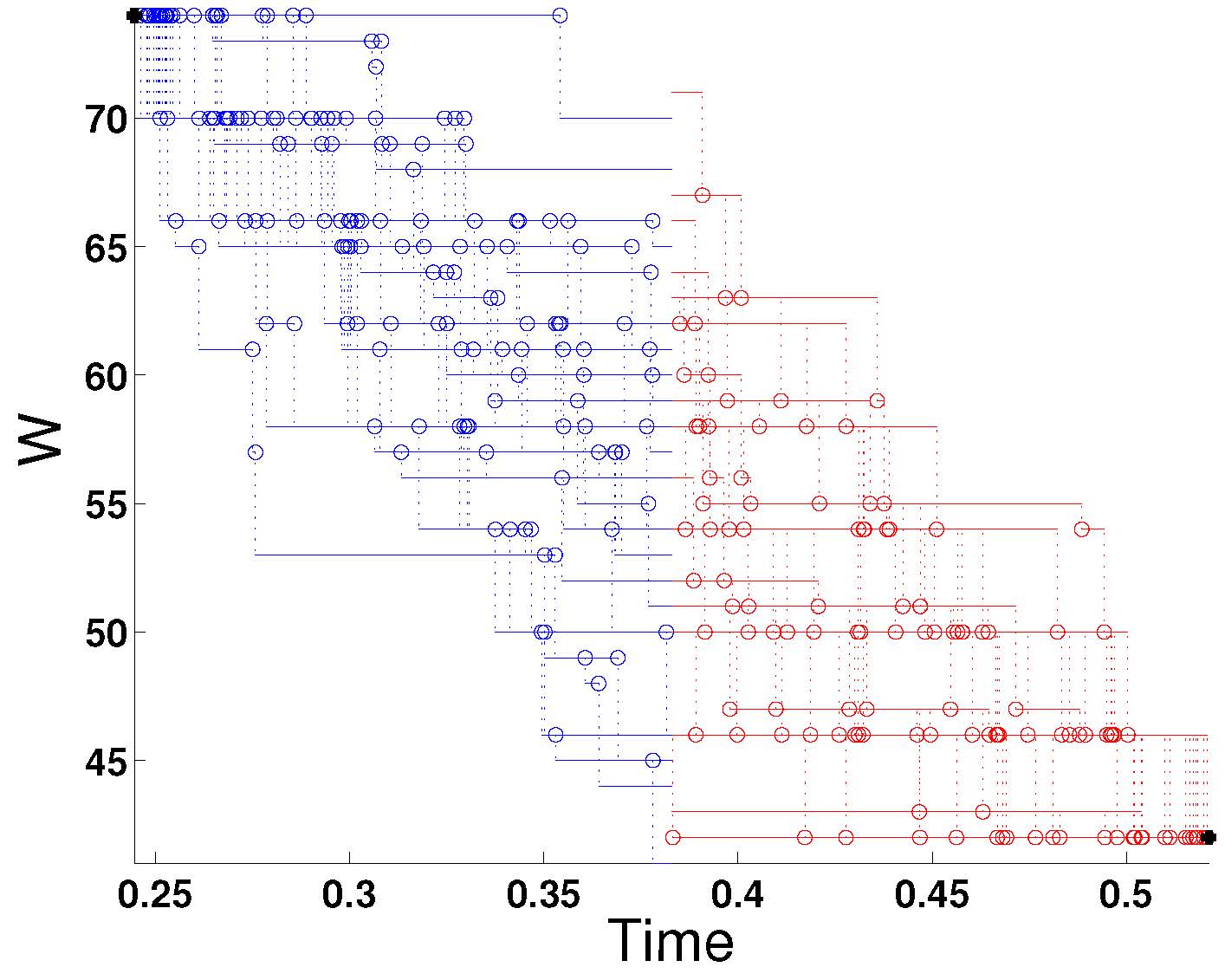
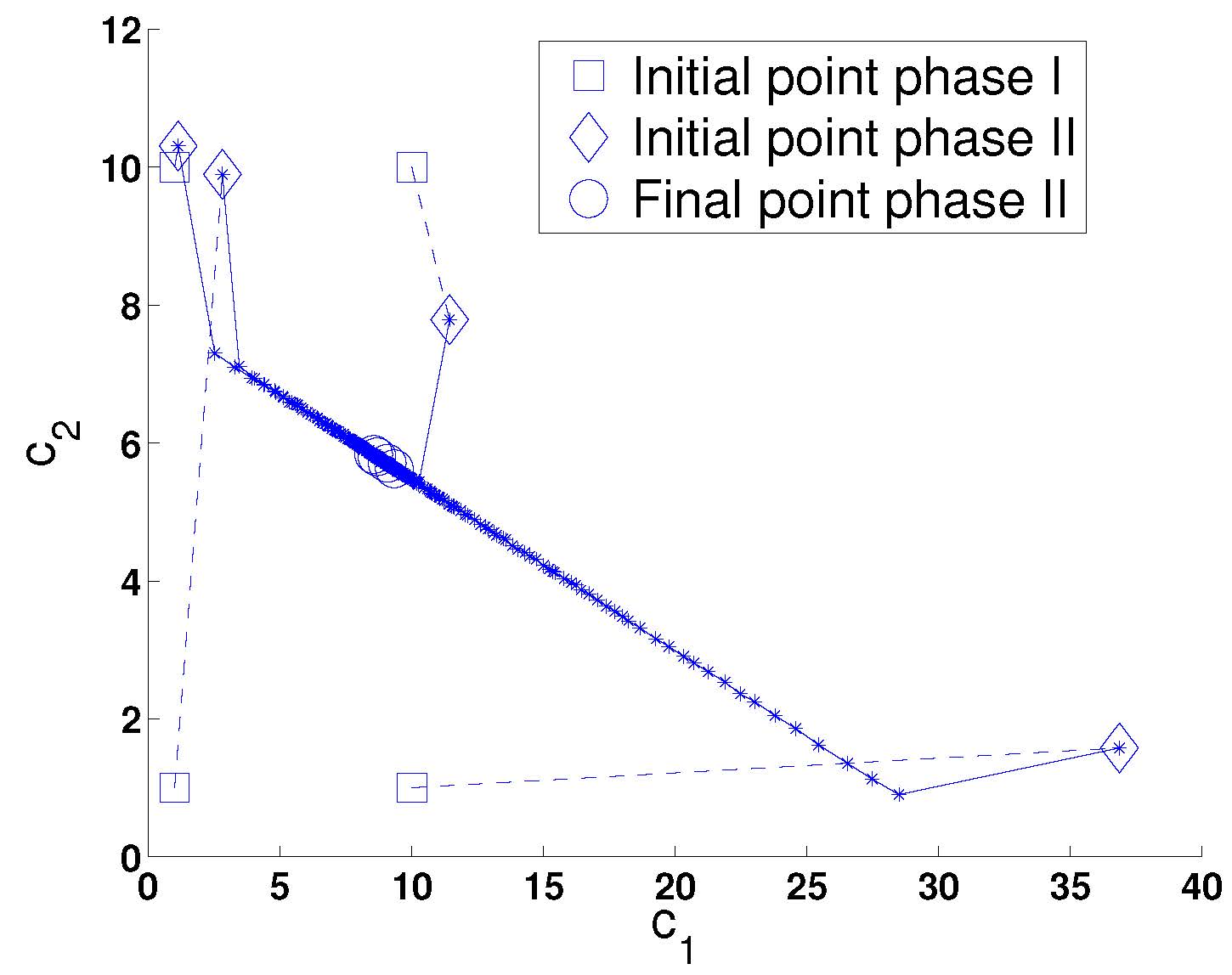