Stochastic differential equations (SDEs), both ordinary time-dependent equations and partial differential equations with random coefficients, are common mathematical tools to model natural processes with uncertainty. In Monte Carlo methods expected values of quantities of interest of the model are approximated by generating many stochastic outcomes of the SDE, where each realization usually requires numerical approximation of the differential equation. The MLMC method is a general technique to reduce the computational cost of Monte Carlo approximations which combines stochastic simulations with differing levels of resolution; see http://people.maths.ox.ac.uk/gilesm/mlmc_community.html
We work on MLMC methods in the context of goal oriented adaptive methods, where the degrees of freedom for each outcome of the SDE are chosen adaptively to compute a given quantity of interest to a specified accuracy at a minimal cost. We apply the same type of adaptive algorithm for both time-dependent Ito SDEs and elliptic partial differential equations with random coefficients.
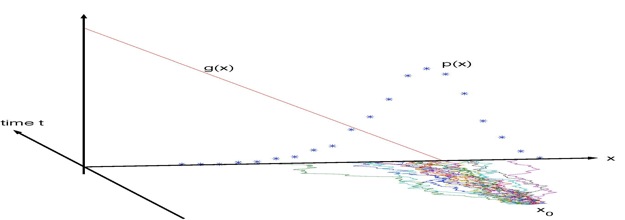